Sector Insights
Providing cutting edge analytics to fulfill the environmental assessment and monitoring requirements of our clients.
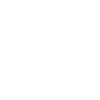
Forestry - arboSense
- Forest extent and type
- Leaf area index (LAI)
- Tree canopy density (TCD)
- Vegetation canopy height (VH)
- Above ground biomass (AGB)
- Canopy disturbance and recovery assessment
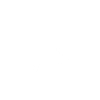
Wetlands
- Wetland class and form classification
- Wetland permanence assessment
- Wetland wetness trend monitoring
- Watershed characterization
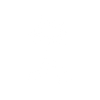
Coastal Management
- Mangrove extent & change
- Mangrove canopy density & change
- Aquaculture inventory
- Coastal habitat assessment and monitoring
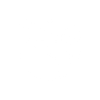
Climate change & nature-based solutions
- Historical baseline assessment
- Habitat degradation and disturbance monitoring
- Restoration and rehabilitation planning and monitoring
- Measurement, reporting, and verification (MRV) for REDD+
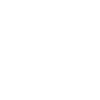
Energy
- Energy corridor monitoring
- Incident response and assessment
- Habitat disturbance and reclamation monitoring
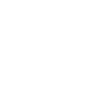
Hazards
- Wildfire – rapid burned area and burn severity mapping (R-BAM)
- Flood – rapid mapping and historical assessment
- Natural disturbances (e.g., insects)